
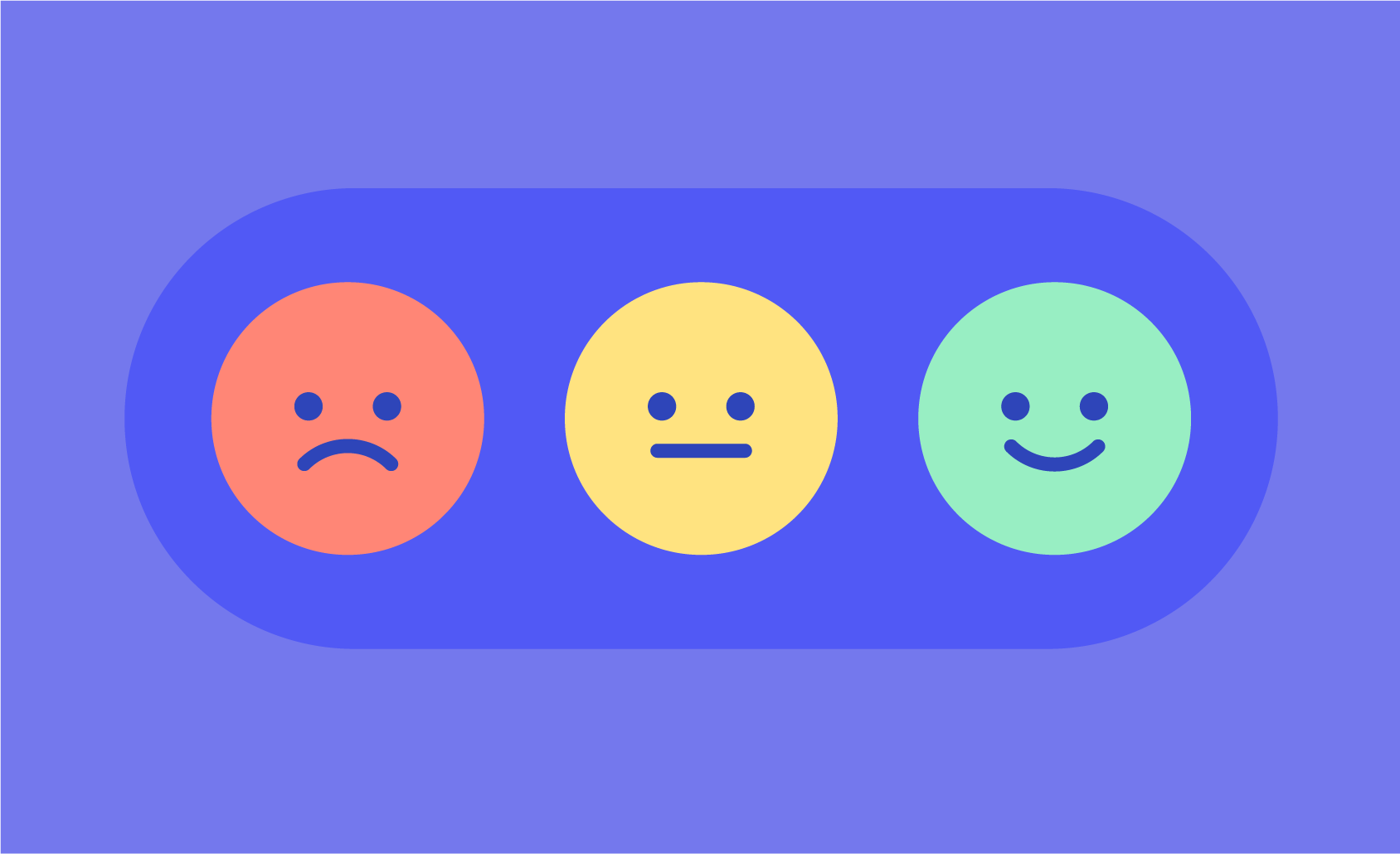
As text analytics tools continue to evolve with AI, sentiment analysis remains a key tenant of any qualitative research project. It cuts to the core of how customers perceive brands, products, and services, summarizing the mood behind consumer feedback and providing valuable insights.
The capabilities of sentiment analysis have been rapidly improving with advancements in machine learning and natural language processing (NLP). While this has made sentiment analysis more widely available and scalable for researchers, it has also brought new challenges. Here, we look at how you can maximize AI technologies and perform effective sentiment analysis that accurately captures the mood of your customers.
1. Find the Right Sentiment Analysis Tool
Every sentiment analysis use case is going to be slightly different. That's because the way people talk varies in different contexts. For example, the way a customer reviews a restaurant will differ from how a voter will discuss an upcoming election. This means there is no one-size-fits-all solution.
It is important to experiment with a wide range of sentiment analysis tools to see which models work best for your text data. Some tools are finely tuned to detect sentiment on social media channels, while others are better suited for surveys. For this reason, finding what works best for your particular needs will ensure you get the greatest return on your investment.
See it in action.
2. Prepare Your Data for Analysis
It goes without saying, but the outcome of your sentiment analysis will only be as good as the data being evaluated. Data preprocessing is a critical step, involving cleaning the data (removing stop words, etc.), tokenization, stemming, handling missing data, converting emojis into words, part-of-speech tagging, and more.
Luckily, much of this process can now be automated thanks to advances in NLP, but it is still important to take a close look at your data before you start testing it. Some common issues to keep an eye out for include;
- multiple words being used to indicate the same thing
- differences in text capitalization
- variations in spelling
- potential loss of nuance due to stemming
Want to see how to do sentiment analysis yourself?
Start a free trial of Displayr.
3. Keep a Human Touch
Sentiment analysis is all about human emotions—and who better to sense-check this than an actual human? AI has given us the ability to perform sentiment analysis on a massive scale, but a level of human intervention is required to interpret and contextualize the insights. Humans play an important role in fine-tuning the analysis model and identifying mistakes in the analysis. Human involvement in sentiment analysis is not just recommended, it's a must. Although AI is rapidly improving, the risk of error is still too high to justify fully automating a project. Keeping humans in the loop will not only enhance the accuracy your project, but it will also improve the model's capabilities moving forward.
Displayr's sentiment analysis tools make it easy for you to categorize text data and understand the mood of customers at scale.
Want to see it yourself? Start a free trial.