
Using Correspondence Analysis to Find Patterns in Tables
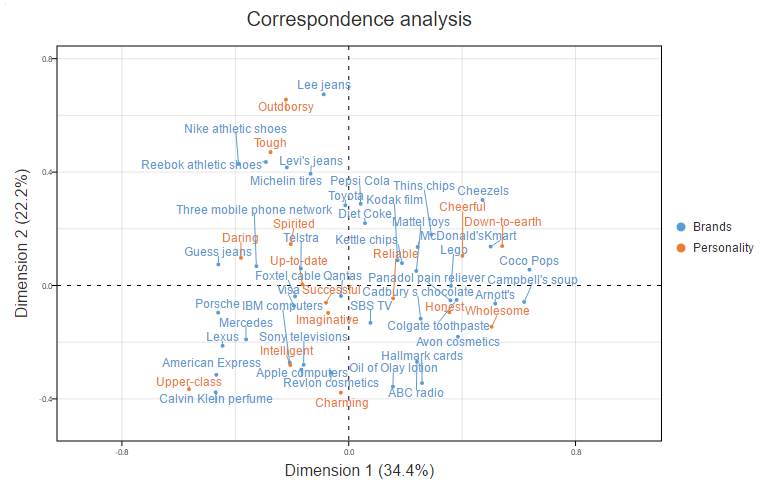
There are lots of great visualizations designed for analyzing big quantities of data. Heatmaps, for example, are super-popular. However, when I am in a rush, my "go to" approach to analyzing big tables is almost always correspondence analysis. Although a bit more technical, it tends to get me to the key insights much faster.
A Correspondence Analysis Example
The data below shows the proportion of people to associate 15 personality attributes with 42 brands. It is too big, making it difficult readily digest.
The scatterplot below shows the results of a correspondence analysis of the same table. Correspondence analysis identifies the main relationships between the rows and columns on a table and plots them on a two-dimensional map. You could have more dimensions, but as computer screens are two-dimensional, they tend not to be so good.
Interpreting Correspondence Analysis
The chart above is much simpler to digest than the whole table. At the bottom-left, we can see that that Calvin Klein, American Express, Apple, and Lexus are Upper-class. Porsche mixes Upper-class and Daring. At the top-left, we can see that Tough is shared by Nike, Reebok, Levi's and Michelin, which also are a bit Outdoorsy.
One key tip if you are new to correspondence analysis: the closer anything is to the middle of the map, the less distinct it is. Thus, on this map, we can see that Qantas is poorly described by any of the personality attributes. Similarly, Successful and Imaginative are personality attributes that are not good differentiators between the brands.
We can also see that a continuum of sorts is evident in the data. It goes from Upper-class and Intelligent at the bottom-left, through to Cheerful and Down-to-earth at the top-right.
As is always the case when we fit a model to data, there is no free lunch. Correspondence analysis just summarizes the data. Like many summaries, it can be superficial and at times misleading. For this reason, I always check that any key conclusions that I draw from a correspondence analysis are also clearly visible in the original data table or a heatmap.
You can create your own correspondence analysis by using the template above.