
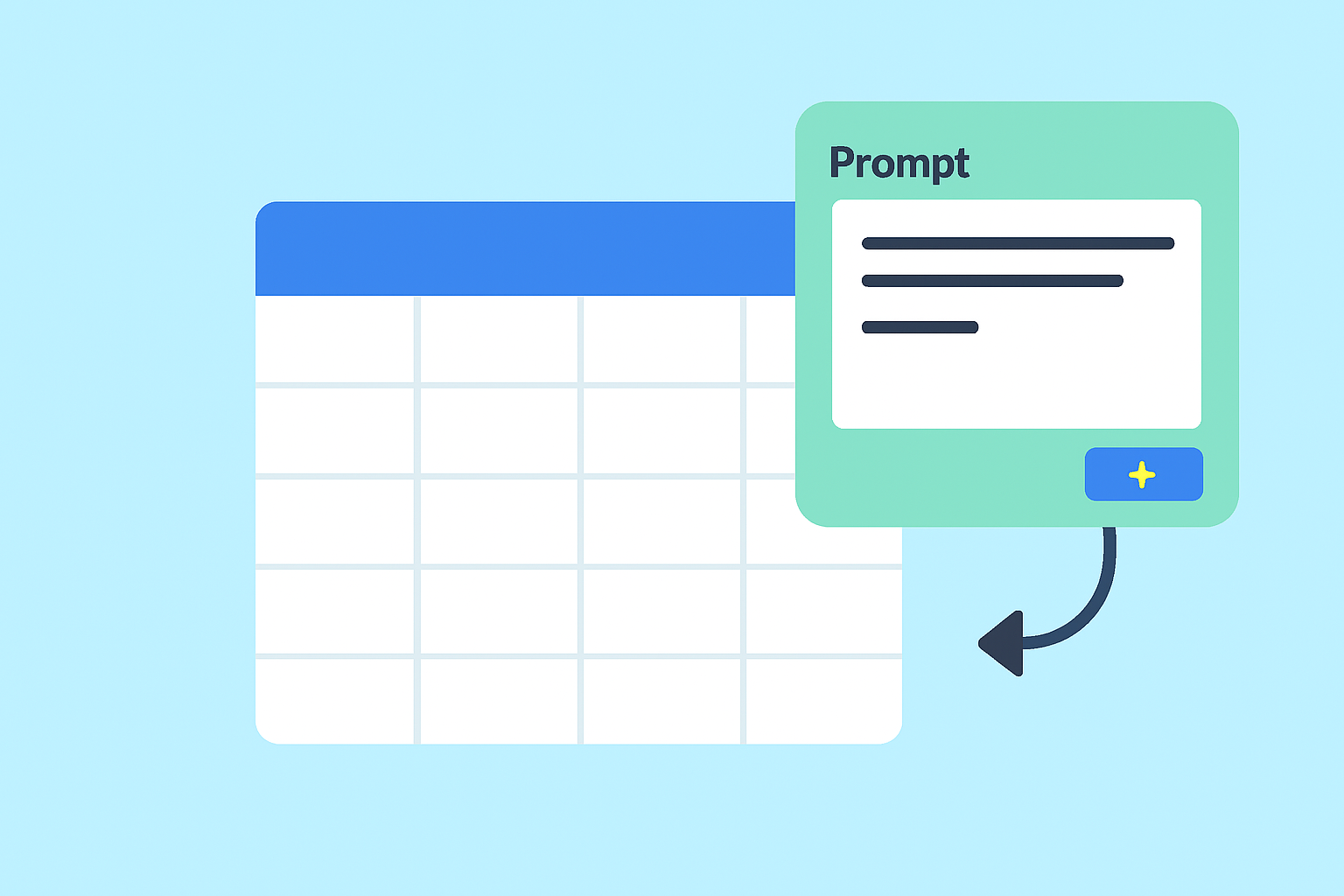
As generative AI tools become more pervasive, writing clear and direct prompts has emerged as a critical skill. Prompts are instructions or queries that describe the task the AI should perform. For best results, they need to be clear, specific, and concise, providing context when necessary.
AI text analytics prompts for market research
If you're a market researcher, high-quality prompt engineering is like having a research assistant by your side. With the right tools, you can use AI to access critical information from outside your actual dataset in seconds.
For example, if you were using Displayr to analyze text indicating the popularity of individual SKUs from a grocery store, you could ask your AI assistant to categorize items based on whether they contain sugar or not.
Again, the key here is to provide a clear and specific prompt. Rather than simply saying, 'categorize items based on whether they contain sugar or not', a more effective prompt is:
Assign all the SKUs into the following categories:
Contains sugar
Does not contain sugar
In terms of text analysis, this gives you the ability to categorize your data in new ways. It also serves as a way to generate insights from a dataset that might have previously seemed uninteresting. As is always the case with AI, it is important to look for any accuracy errors and double-check that the text has been categorized correctly.
Assign numeric values
Creative prompt engineering can also allow you to assign numeric values to text quickly and easily. One of the most common forms of text analysis is calculating sentiment score. Sentiment analysis is a way to gain a snapshot of written tone and develop ideas to improve services and products. It assigns a value of +1 for positive terms and -1 for negative terms.
But the bare bones of sentiment analysis - that is, assigning a numeric value to text - can be extended into a variety of use cases. For example, a hotel could investigate the number of different factors that come up in their reviews to see if there is any correlation with sentiment. In Displayr, you could ask AI:
'Please give a score based on the number of different aspects of the hotel that are discussed, giving a score of 1 if they only discussed one thing, 2 if they discussed 2 things, etc.'
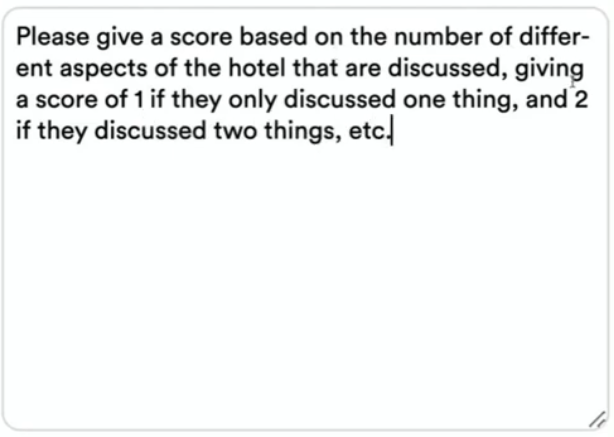
You can use prompt engineering to quickly find the sentiment of your text.
This is another example of using imaginative prompt engineering to gain deeper insight faster. Of course, you could manually set up this type of analysis. However, this would take time and may not be worthwhile.
Ready to analyze your text?
Start a free trial of Displayr.
Prompt engineering best practices
As mentioned previously, writing prompts that are clear, specific and concise will produce the best results. However, when writing prompts for something as specific as performing text analysis in Displayr (or any other tool) there are additional best practices to keep in mind.
First, providing an example is a great way to increase the chance of success when performing more complex tasks. The hotel review use case given above could be improved with a specific example, as shown below.
'Please give a score based on the number of different aspects of the hotel that are discussed, giving a score of 1 if they only discussed one thing, 2 if they discussed 2 things, etc. For example, "very affordable" would be given a score of 1, and "the environment, facilities, etc are all very good" would be given a score of 2.
Another way to improve your prompt engineering is to break up complex requests into sequential steps. This is sometimes referred to as prompt chaining. It gives you greater control over the output, as you have greater visibility and control over each phase, while the AI model can 'think' through the process. Again using the hotel use case as an example, the prompt chaining would look like:
Analyze the hotel reviews by completing the following steps.
- Translate the text into English.
- Perform a sentiment analysis of the reviews with a value of +1 for positive terms and -1 for negative terms.
- Give a score based on the number of different aspects of the hotel that are discussed, giving a score of 1 if they only discussed one thing, 2 if they discussed 2 things.
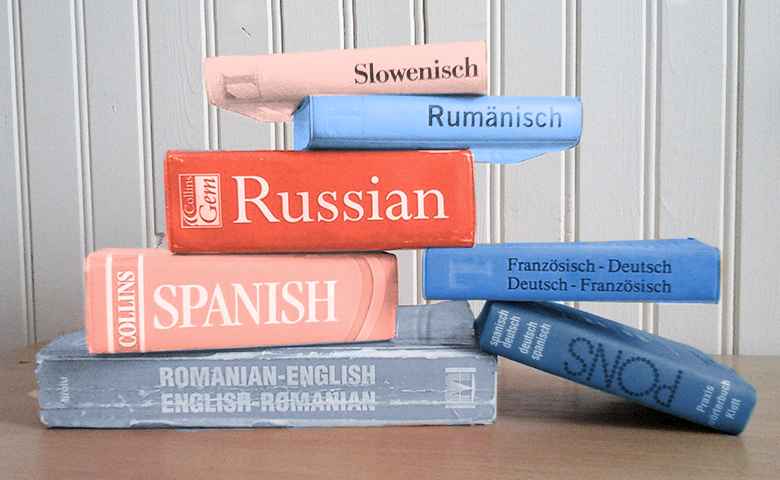
Translate text from whatever language you are working in.
Transform your text data into actionable insights with Displayr’s AI-powered text analysis.