
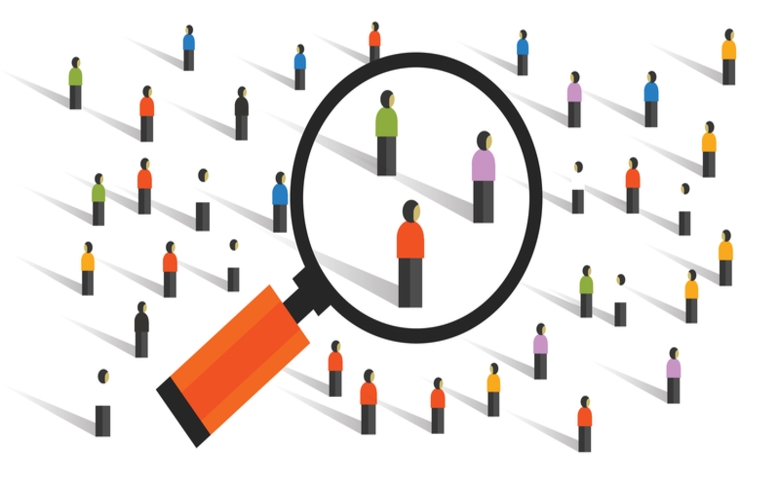
In this post, I'll explain what random sampling is and the different types of random sampling you might come across and an alternative to the random sampling that you may want to consider.
What is random sampling?
When carrying out a survey, it would be impractical to study a whole population. Sampling is a method that allows researchers to infer information about a population based on results from a subset of the population. It is important to ensure that the individuals selected are representative of the whole population.
There are several different sampling techniques available that can be grouped into two categories as probability sampling, and non-probability sampling. The difference between the two techniques is whether the sample is selected based on randomization or not.
In probability sampling, alternatively knows as random sampling, you start with a complete sample frame of all eligible individuals that have an equal chance to be part of the selected sample. The selection must occur in a 'random' way, meaning that they do not differ in any significant way from observations not sampled. It is typically assumed that statistical tests contain data that has been obtained through random sampling. For example, exit polls from voters that aim to predict the likely results of an election.
The following random sampling techniques will be discussed: simple random sampling, stratified sampling, cluster sampling, and multi-stage sampling. Non-random sampling techniques are often referred to as convenience sampling.
Simple random sampling
Simple random sampling is the most straightforward approach to getting a random sample. It involves picking the desired sample size and selecting observations from a population in such a way that each observation has an equal chance of selection until the desired sample size is achieved. For example, a random selection of 20 students from a class of 50 students gives a probability of selection being 1/50.
Stratified random sampling
This technique divides the elements of the population into key subgroups or strata. The elements are randomly selected from each of these strata. For example, males under 30, females under 30, males 30 or over, and females 30 or over. Say you want to achieve a sample size of 200, then you can pick samples of 50 from each stratum. The required sample size for each stratum will be designed either to match the known population proportions or to over-represent key subgroups of interest. We need to have prior information about the population to create subgroups. The main benefit of stratified sampling over simple random sampling is making sure that you have good sample sizes in key subgroups.
Cluster sampling
Similar to stratified random sampling, cluster sampling divides the sample into a large number of subgroups. Then some of these subgroups are selected at random, and simple random samples are then collected within these subgroups. These subgroups are called clusters.
Typically, the purpose of cluster sampling is to reduce the costs of data collection. This is achieved by defining clusters according to the ease of access (e.g., a suburb may be a cluster if door-to-door sampling or a household may be a cluster if phone interviewing).
Multi-stage sampling
Multi-stage sampling is a combination of one or more of the techniques described above. The population is divided into multiple clusters and then these clusters are further divided and grouped into various subgroups (strata) based on similarity. One or more clusters can be randomly selected from each stratum. This process continues until the cluster cannot be divided any further
Alternatives to random sampling
Convenience sampling refers to approaches where considerations of simplicity rather than randomness determine which observations are selected in a sample. Here the samples are selected based on availability. When the availability of samples is rare, convenience samples are selected. This is used generally during the initial stages of a survey and is quick and easy to deliver results.