
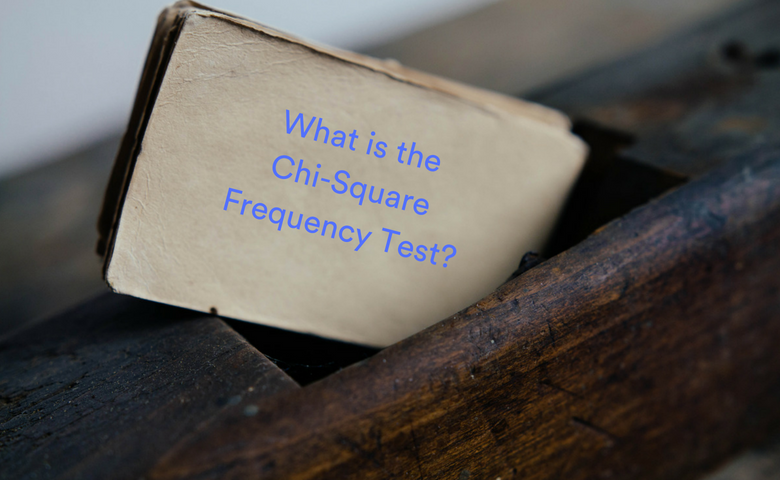
TL;DR
The chi-square frequency test is a statistical test that determines whether the observed number of people with different values of a variable aligns with expectations. It is commonly used to assess if the preference for one option over others is statistically significant (e.g., determining if voting intentions for one candidate are significantly higher than for another in an opinion poll). This test is also referred to as the chi-square goodness-of-fit test.
Example of the chi-square frequency test
A joint The Economist/YouGov poll for early April 2018 found that 41% of registered voters approved of the performance of President Trump, 55% disapproved, and 4% were not sure. The survey totaled 1,246 registered voters. The question is whether the difference between the 41% and the 55% is likely due to a sampling error, or whether it indicates that the majority of registered voters are dissatisfied with President Trump.
By multiplying the percentages by the sample size and ignoring the people who were not sure, we have observed counts of 511 people disapproving and 685 people approving.
Let's say that the null hypothesis of there being no difference between the proportion of people approving versus disapproving is true. This would mean that the observed difference between 511 and 685 is due to sampling error. In this case, we would expect that of the 511 + 865 = 1196 people, 50% = 598 would approve and the other 598 would disapprove. Thus, if the null hypothesis is true, our expected counts are 598 and 598 for approval and disapproval, respectively.
Sampling error
The extent to which the observed counts differ from expected counts reflects sampling error. You can calculate the chi-square statistic, which summarizes the overall extent of the sampling error. We start by calculating the cell chi-square value. Where O is the observed value in a cell, E is the expected value, the formula is (O – E)²/E. For the first of our two cells, we get (511 - 598)²/598 = 12.657. We need to repeat this calculation for all the cells in the table and then sum these up. In this example, we also get 12.657 in the second cell, so the total, which is called the test statistic, is 25.314.
One we know the value of the test statistic, the next step if to compute the degrees of freedom, which is computed as Number of categories – 1, which is in our example is 2 – 1 = 1.
As this is a chi-square test, we can look up the test statistic and the degrees of freedom for the chi-square distribution, which is 1 for this example, and we get a p-value of 0.0000005. This is well below the standard cutoff value of 0.05, which tells us that we can reject the null hypothesis that sampling error explains the difference between the approvers and non-approvers (i.e., the conclusion is that significantly more people disapprove than approve of the performance of President Trump at the time the data was surveyed).
Modifying the test for other data
This example applied the test to two categories (approve and not approve). You can compute this test for more categories beyond the test statistic described above.
If we had some other null hypothesis, such as that one-third of people approve, we would compute the expected counts by multiplying the total sample size by whatever percentages are appropriate.
Acknowledgments
You can find the data we used here.
Discover more key terms in Displayr's "What is" series!