MaxDiff.
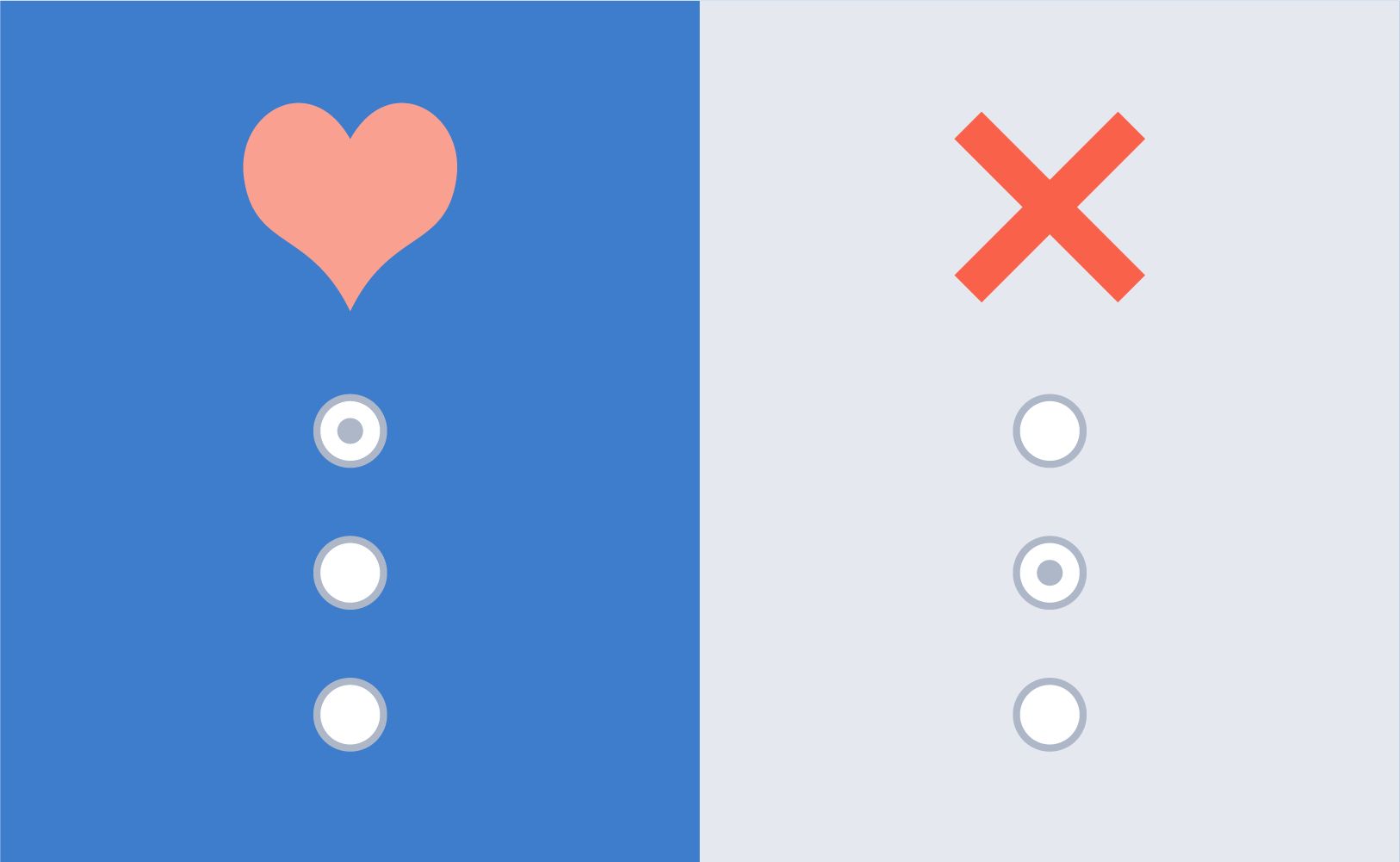
MaxDiff is a survey research technique for working out relative preferences for multiple items. It is also known as maximum difference or best-worst scaling.
Continue reading
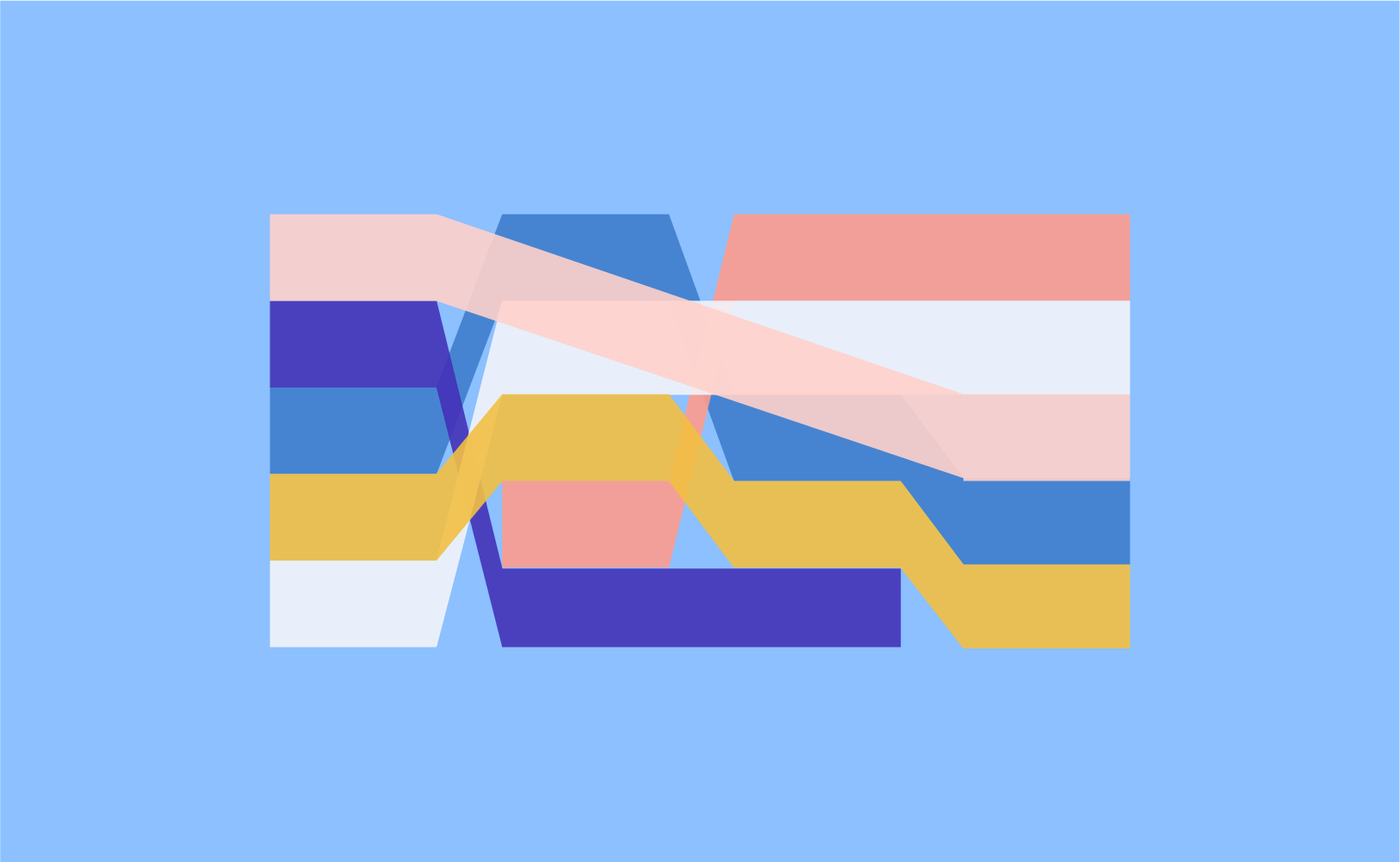
This is a guide for everything you need to know about MaxDiff. It covers the “what is?” and the “how to…” of different approaches to…
Continue reading
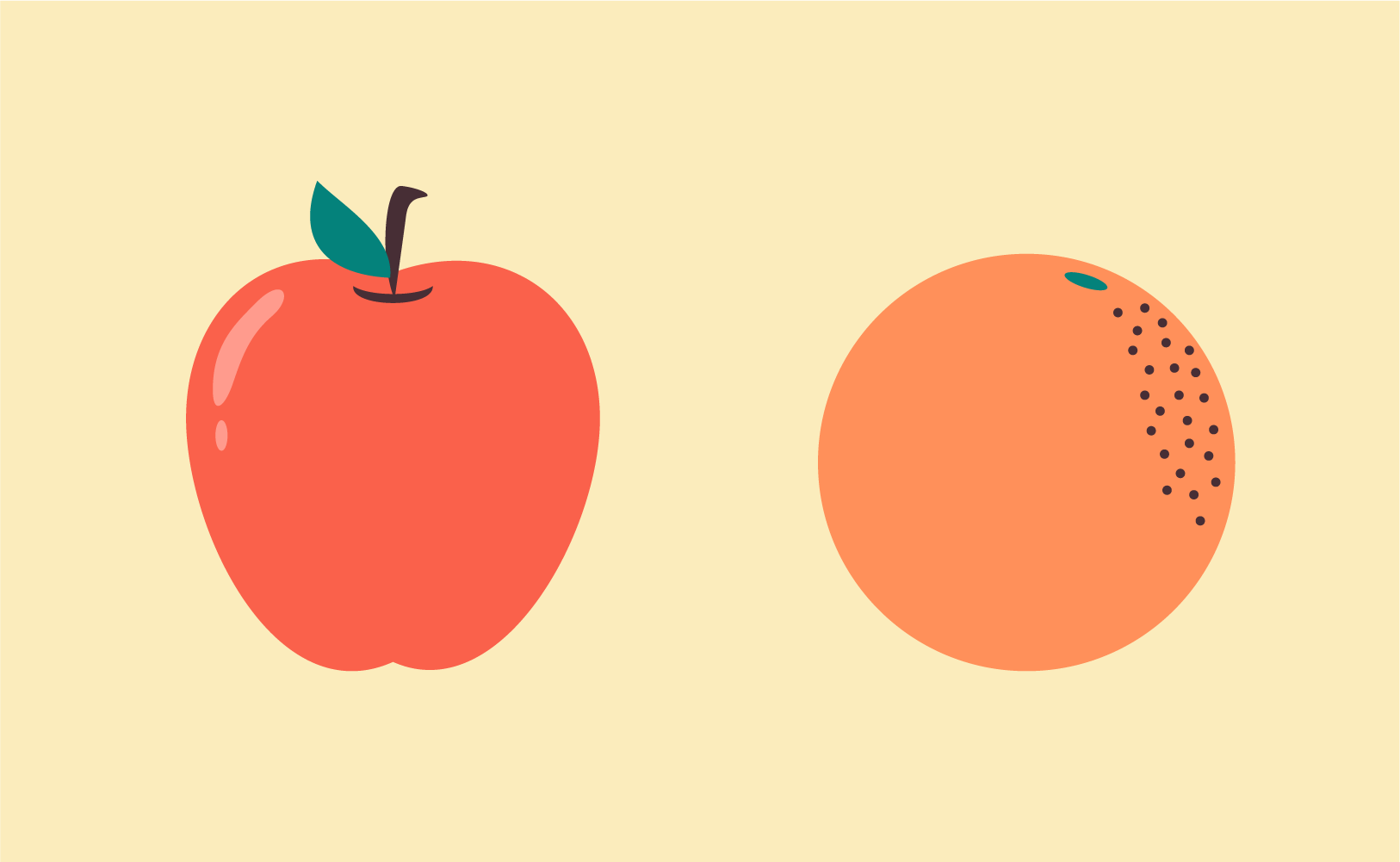
Different models There are lots of different statistical models that you can use to compute MaxDiff. Some of these get different results from Sawtooth simply…
Continue reading
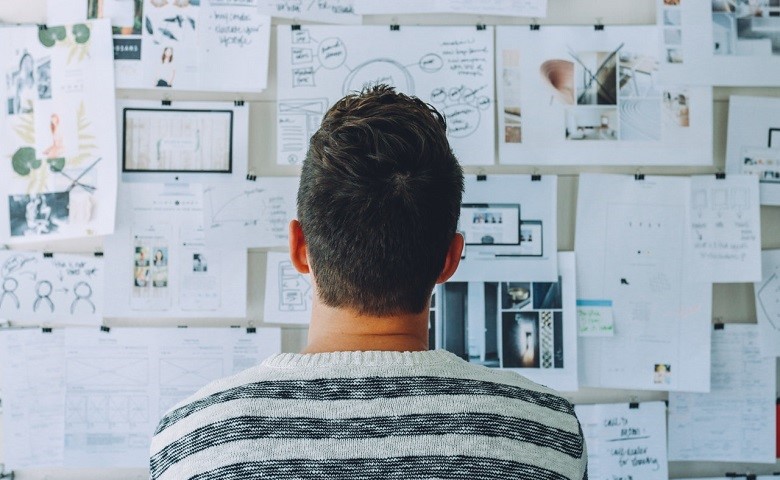
There are a variety of different models available in Displayr to perform MaxDiff analysis. I'll show you how to easily compare the models.
Continue reading
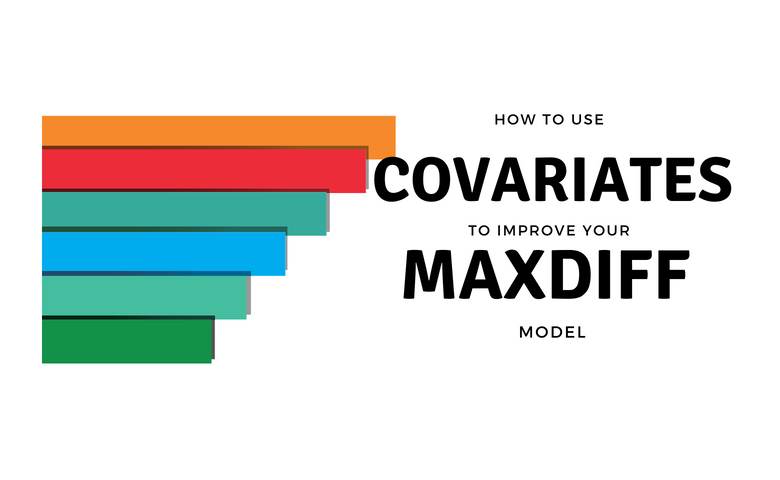
Ready to improve the accuracy of your MaxDiff model by including covariates? I'll show how using Hierarchical Bayes for MaxDiff.
Continue reading
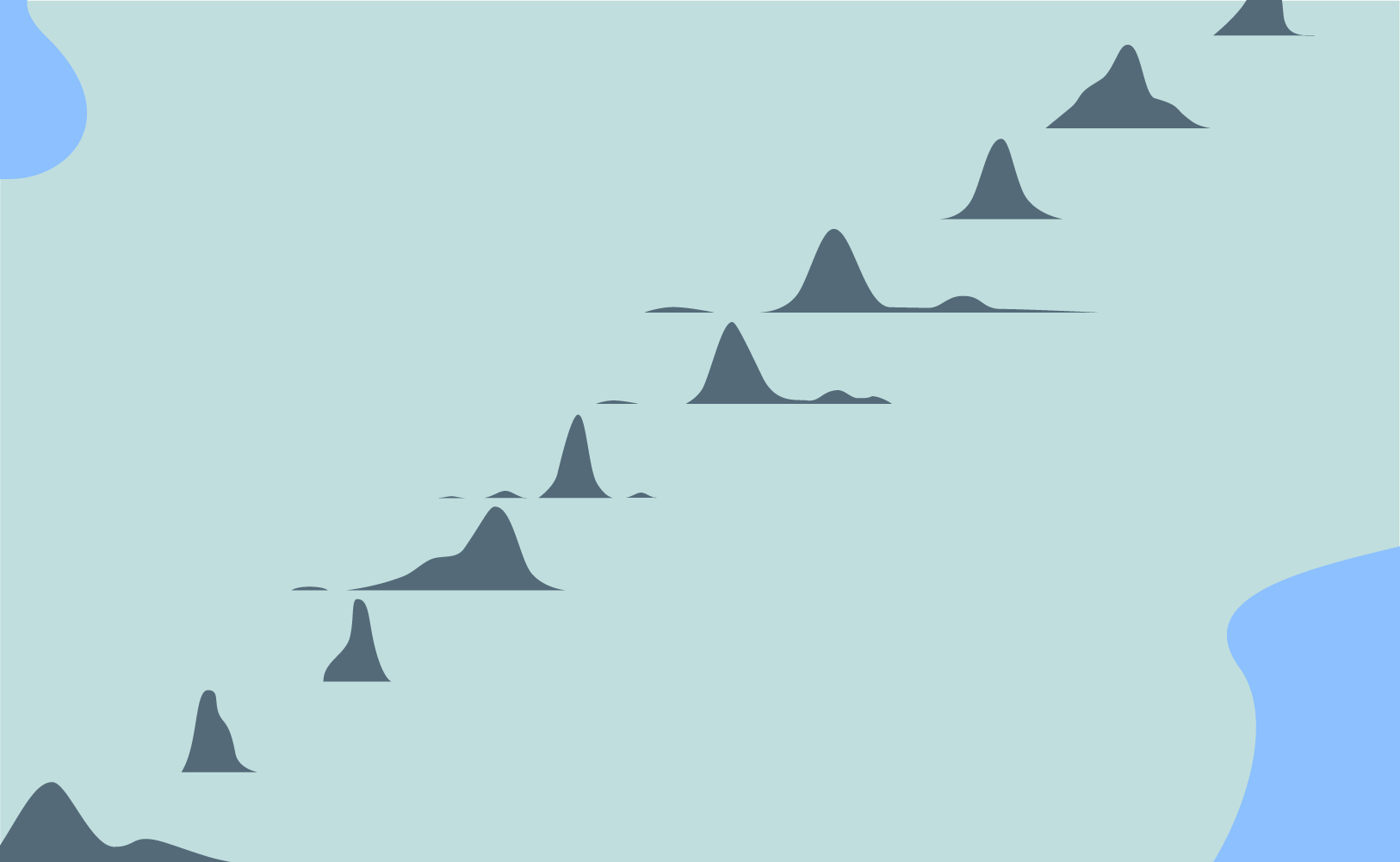
In this post I explore the implications of using hierarchical Bayes versus using latent class analyis for data which contains segments.
Continue reading
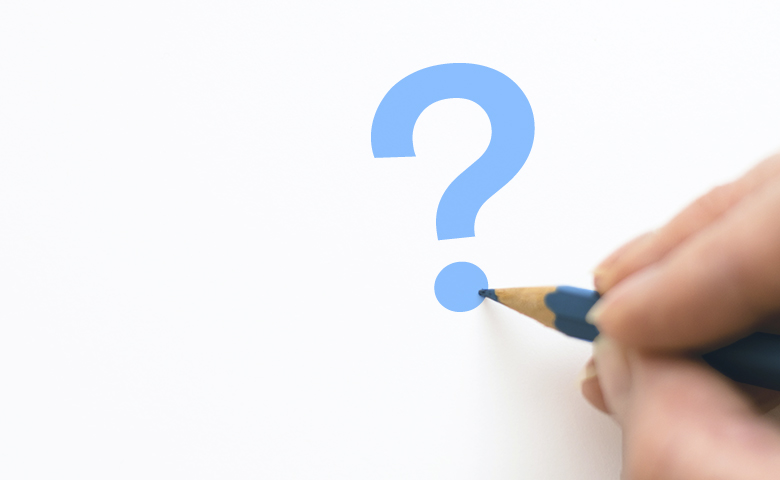
Pairwise balanced MaxDiff designs are an improvement upon multiple version designs that have been randomly permutated. I'll show you how you can create your own...
Continue reading
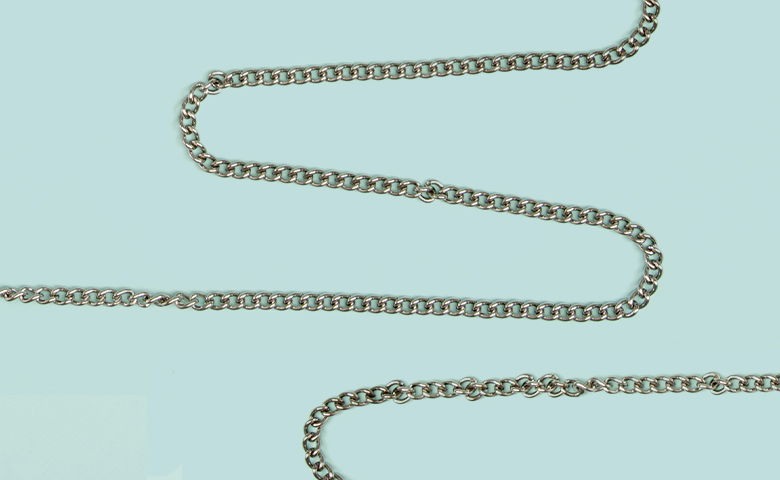
Hierarchical Bayes is an approach to modelling MaxDiff data which can provide better predictive accuracy than other approaches (like latent class analysis).
Continue reading
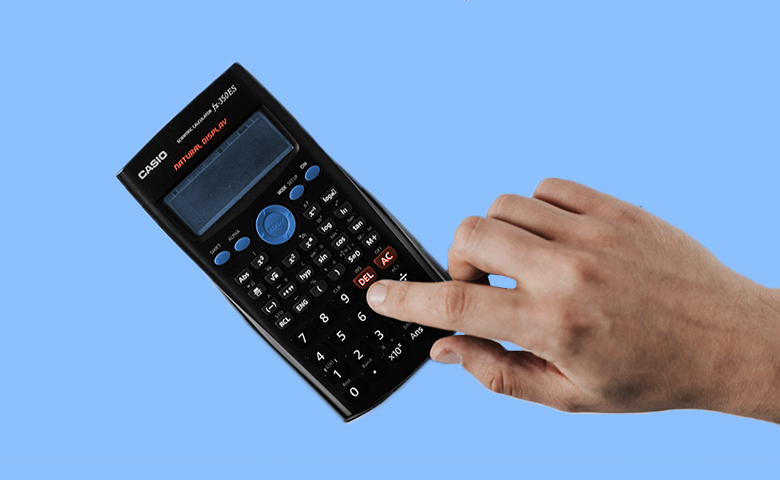
Getting started Your MaxDiff data needs to be in the same format as the technology companies dataset used in previous blog posts on MaxDiff such…
Continue reading
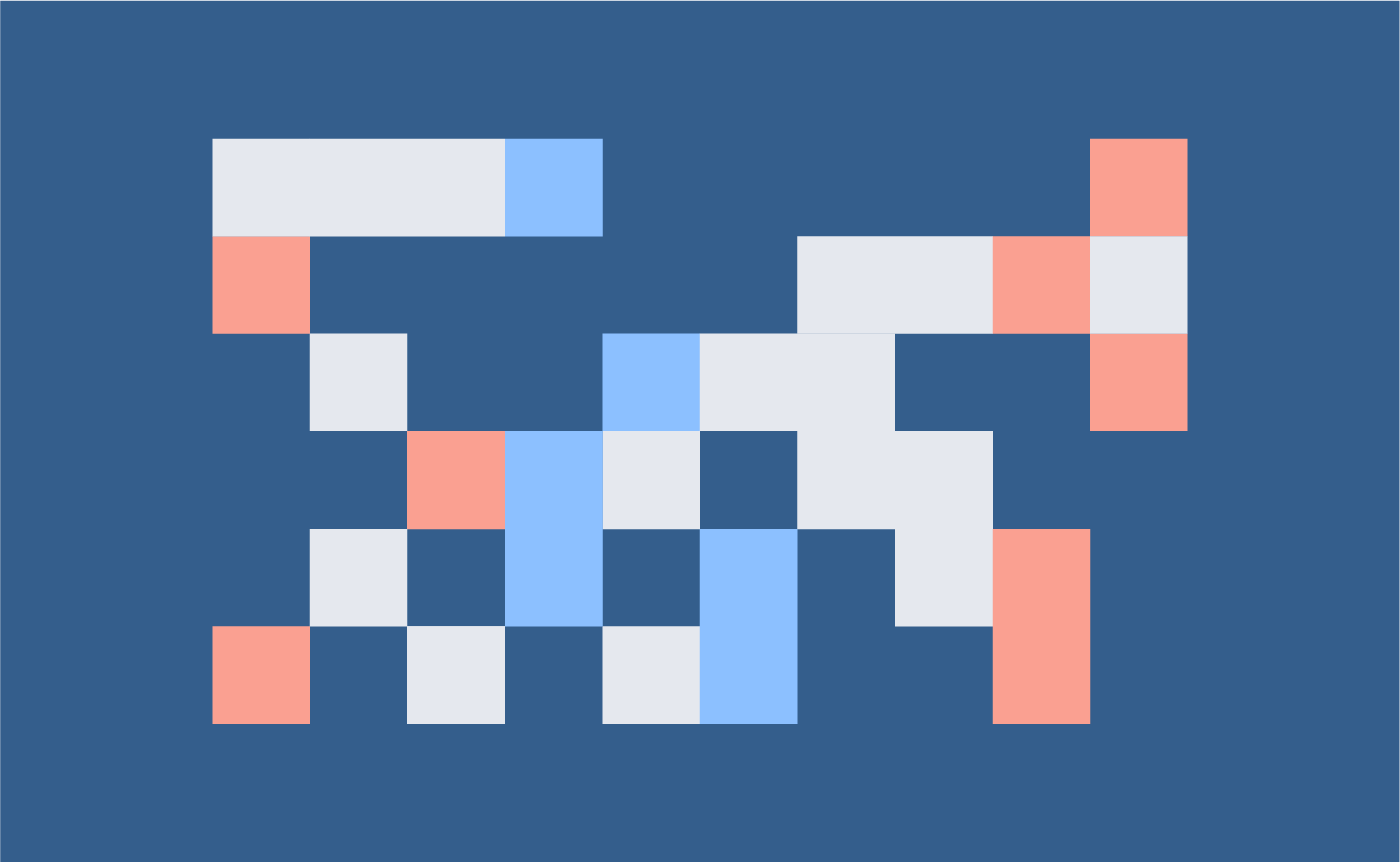
This article compares two ways to analyze Max Diff data - Tricked Logit and Rank-Ordered Logit with Ties - for latent class analysis or Hierarchical Bayes
Continue reading
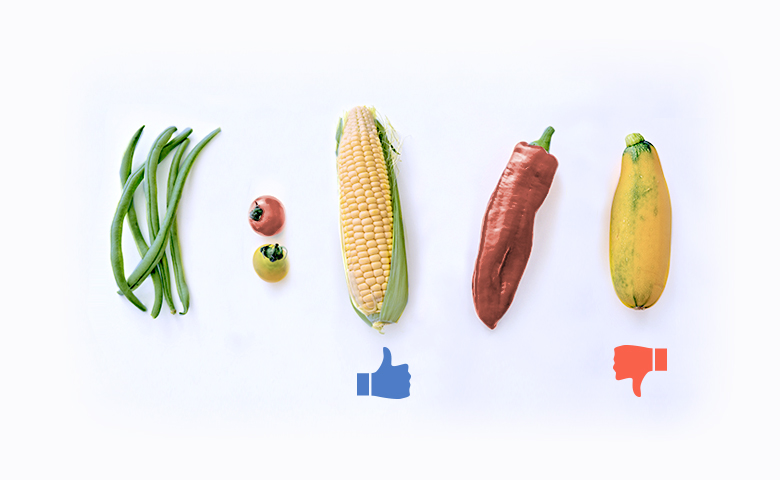
MaxDiff analysis is an advanced technique that can be run by any good quant researcher. Here are 11 tips to help people relatively new to the field DIY.
Continue reading
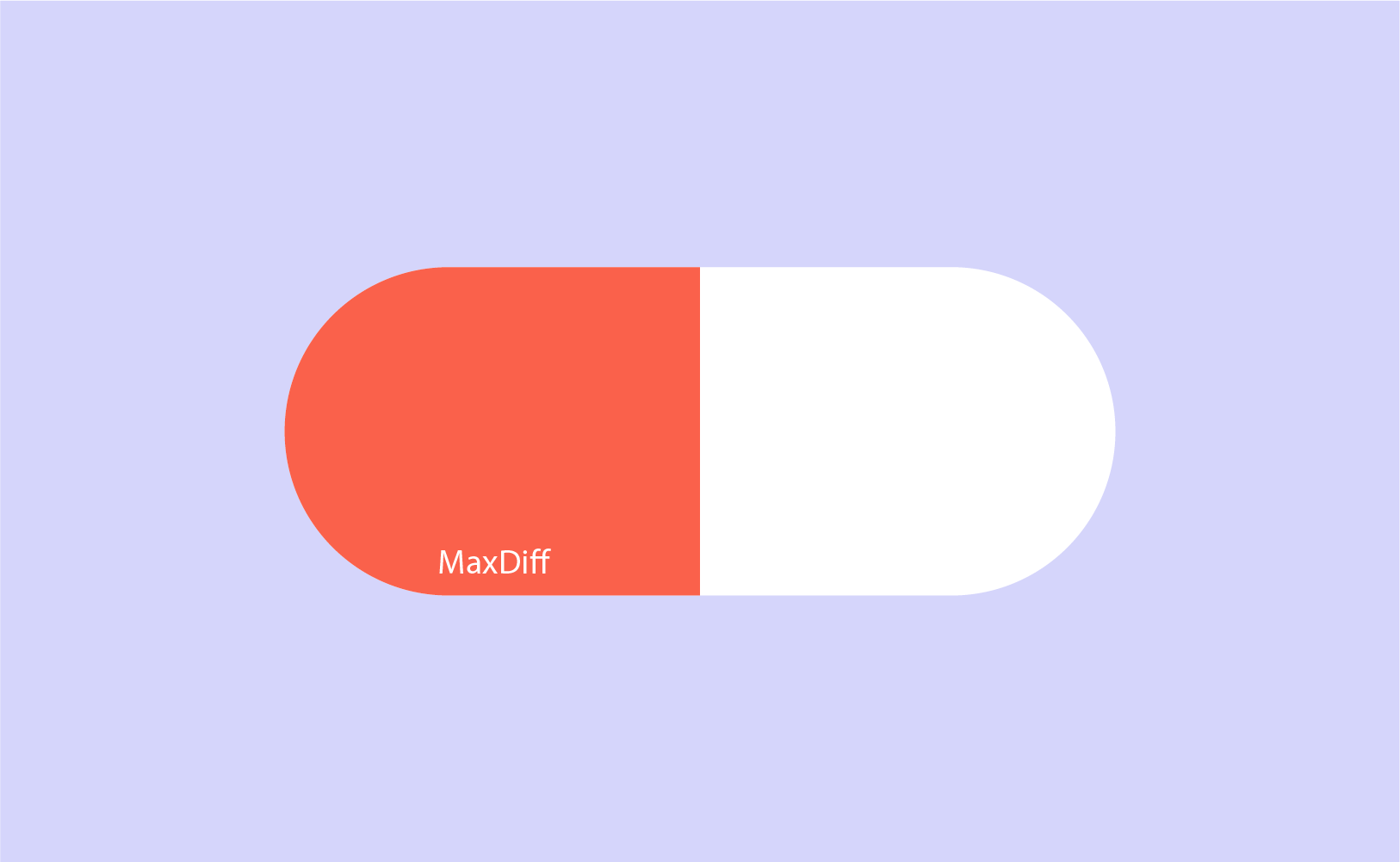
Experimental designs can be scary. Small mistakes ruin everything. I explain the process I use when doing a rough-and-ready check of an experimental design.
Continue reading
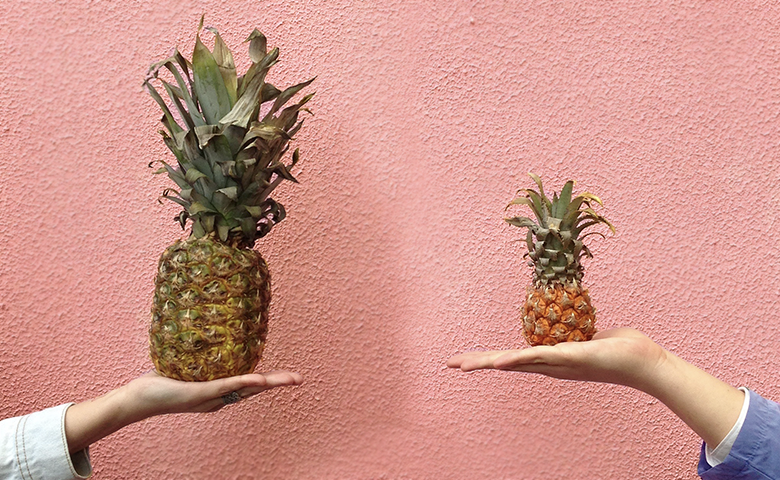
This post compares various approaches to analyzing MaxDiff data using a method known as cross-validation. Before you read this post, make sure you first read How MaxDiff…
Continue reading
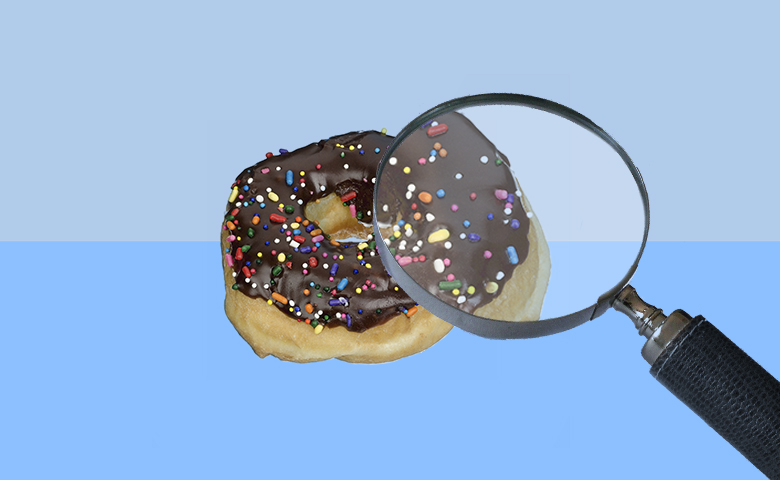
This post discusses a number of options that are available in Displayr for analyzing data from MaxDiff experiments. For a more detailed explanation of how…
Continue reading
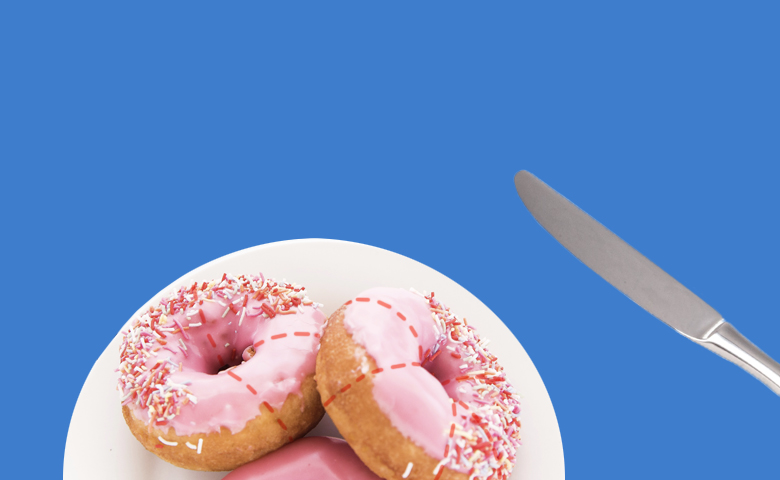
This post explains the basic mechanics of how preferences can be measured using the data collected in a MaxDiff experiment.
Continue reading
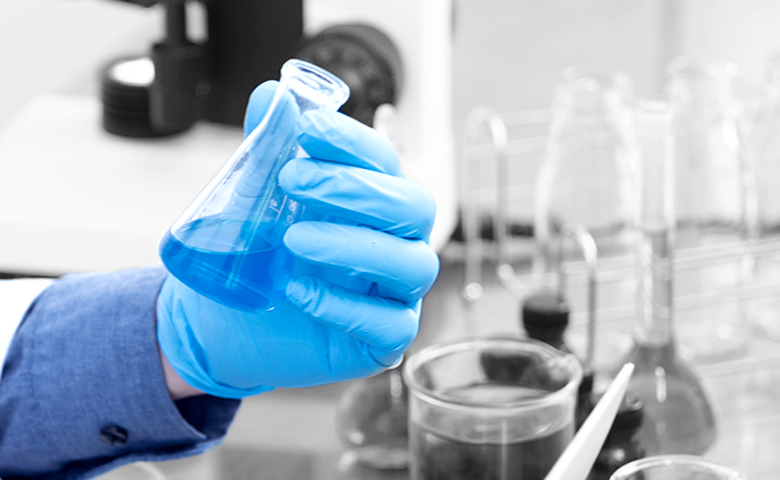
Creating the experimental design for a max-diff experiment is easy in Displayr. This post describes how you can create and check the design yourself.
Continue reading
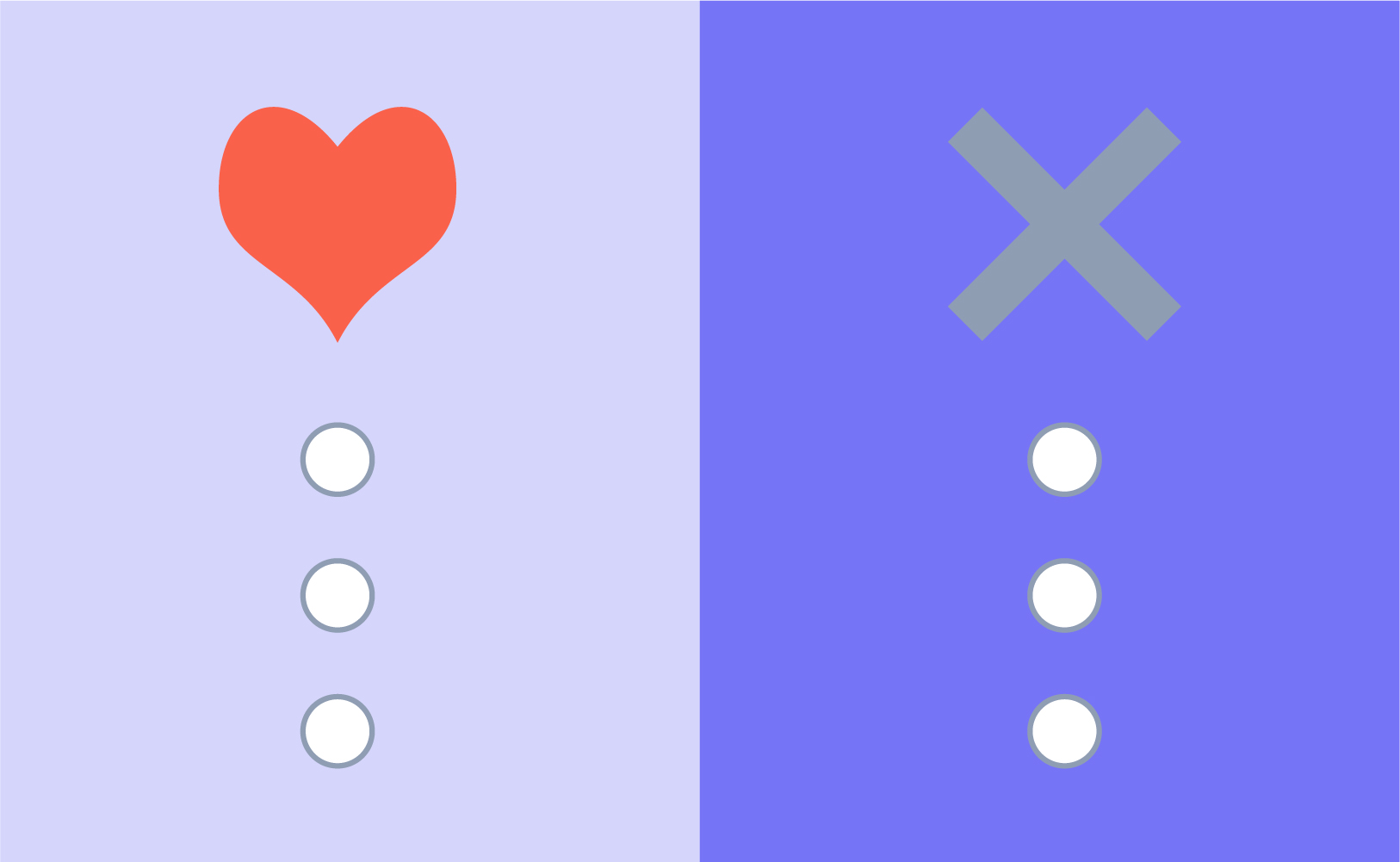
MaxDiff analysis is a technique for measuring relative preferences. This post introduces the four stages of a MaxDiff analysis.
Continue reading